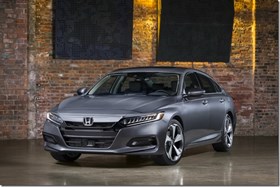
AD&P BLOG
First Look at the 2018 Honda Accord
By: Gary S. Vasilash – July 14, 2017 at 12:00 PM
âWe are redefining the Honda Accord for a new generation of buyers by bringing something unexpected that challenges the idea of what a mainstream sedan can be.â Thatâs Jeff Conrad, senior vice president of the Automobile Div. of American Honda Motor Co., Inc., talking about the 10th generation, 2018 Accord that was unveiled today in Detroit and which will launch this fall.
Related Articles

AD&P FEATURE
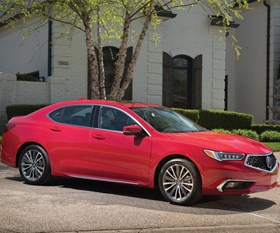
AUTOMOTIVE MANAGEMENT
TLX Reset
For the 2018 model, Acura designers and engineers made changes to the sport sedan that go beyond a typical refresh. Hereâs why and how.
July 14, 2017 – Gary S. Vasilash

THE LATEST INSTALLMENT
AAH #385 – Tearing into the Chevy Bolt EV. TOPIC: Chevrolet Bolt EV. SPECIAL GUEST: Sandy Munro, Munro & Associates. NEWS: Tearing into the Chevy Bolt EV; Doctor Data. Vehicle Walkaround Feature: Get a peek at the new Hyundai Sonata with Chris Chapman, Chief Designer, Hyundai Design Center. PANEL: John McElroy, Autoline.tv; Gary Vasilash, Automotive Design & Production; David Welch, Bloomberg

AUTOBEATDAILY.COM
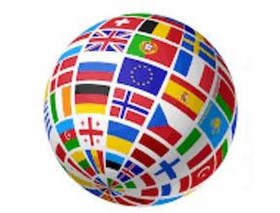
FEATURED
New-Car Deliveries in Europe Climb 2%
Passenger car sales across Europe rose 2% to 1.54 million units in June, according to trade group ACEA. Volume for the first half of 2017 advanced 5% to 8.46 million vehicles.